Channeling the Power of Generative Health AI: Implications for Health Care, Research, and Governance (Part I)
Medicine is one of humanity’s greatest information-processing challenges. Understanding and repairing the human body requires synthesizing vast amounts of interconnected knowledge and information while making high-stakes decisions.
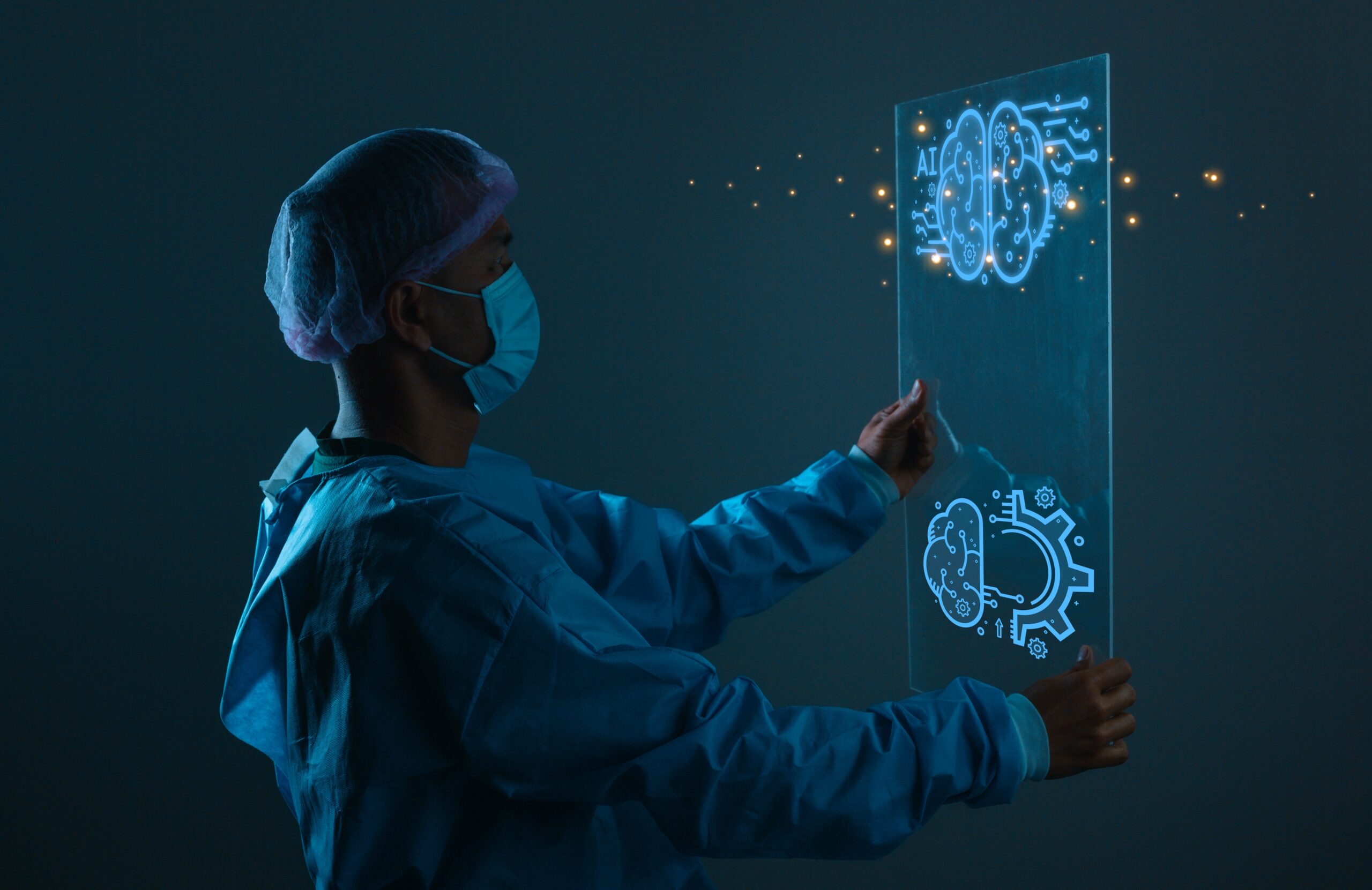
Medicine is one of humanity’s greatest information-processing challenges. Understanding and repairing the human body requires synthesizing vast amounts of interconnected knowledge and information while making high-stakes decisions. Many persistent challenges in medical ethics, human rights, and health care governance can be analyzed as information processing bottlenecks: for instance, the slow evaluations of institutional review boards, systemic flaws in clinical trial design, and the difficulties in keeping up with rapidly expanding medical literature.
Recent breakthroughs in generative AI models suggest new ways of addressing these bottlenecks. When OpenAI released GPT-4 in 2023, its performance was so remarkable that Microsoft researchers declared it exhibited ‘sparks of artificial general intelligence’. Yet, when subsequent improvements seemed to hit a wall, focusing on specialized capabilities—such as GPT-4o’s multimodality and GPT-o1’s chain-of-thought reasoning—many experts concluded that the returns from brute-force scaling were diminishing. These limitations stemmed not only from the soaring compute costs, reaching hundreds of millions of dollars, but also from apparent limits of high-quality training data available on the Internet.
This made the recent emergence of DeepSeek-R1 particularly shocking. Developed at a fraction of the cost of industry leaders, this open-source breakthrough still delivers state-of-the-art performance. This impact was immediate—reportedly wiping out more than $1 trillion in market capitalization across AI companies—and its implications extend far beyond technology, reaching into medical research, health care policy, and ethical and legal frameworks that govern them.
As AI capabilities continue to advance and become more accessible, they could provide the computational power needed to accelerate medical research, improve decision-making, and tackling some of the most pressing ethical and practical challenges in the field.
To fully grasp the implications of these breakthroughs in generative AI models for medicine, we must first explore how they parallel the evolution of human intelligence itself. This comparison not only highlights the extraordinary potential of AI in medicine but also explains its inherent limitations.
Human and Artificial Intelligence
One influential theory of cognitive evolution suggests that intelligence evolved in a series of breakthroughs. Among the most fundamental was pattern matching—the ability to recognize recurring features in the environment and respond with preset behaviors. While this adaptation was crucial for survival, it limited organisms to responding only to familiar situations, with true adaptation occurring slowly through natural selection. Consider how this mirrors early medical AI systems: Pattern-matching algorithms excel at identifying specific abnormalities in medical images, but struggle with rare or complex cases that don’t fit familiar patterns, or do so for the wrong reasons (i.e., some incidental feature).
A transformative advance for biological intelligence came with the development of simulation capabilities—the ability to internally generate and test possibilities through sophisticated internal models of the world. This enabled organisms not only to predict outcomes before taking action but, more importantly, to evaluate those predictions against complex value systems—seeking beneficial outcomes while avoiding harmful ones. In medicine, this manifests as the clinician’s ability to mentally simulate different treatment approaches, anticipating potential outcomes while weighing various factors, including patient values, risks, and quality of life considerations.
With the advent of generative AI, we are witnessing a breakthrough that mirrors this evolutionary transition from pattern matching to generation. Specifically, we are seeing AI not just identify existing patterns in medical data but actively generate novel hypotheses, or predict protein folding patterns. However, despite these impressive capacities, modern AI systems nevertheless lack two fundamental aspects of human intelligence. These two crucial “missing” features are internal world models for testing predictions, and sophisticated value systems for evaluating outcomes.
Internal world models allow humans to simulate possible outcomes before acting, testing different strategies mentally and adjusting based on experience and context. Sophisticated value systems, on the other hand, provide a framework for assessing whether an outcome is desirable—not just whether it’s technically possible—by factoring in complex social, ethical, and personal considerations. Modern AI systems process information at scales far beyond human capabilities, but they cannot yet fully simulate physical reality or autonomously evaluate the desirability of their outputs.
Moreover, they do not simply replicate human intelligence; they embody a fundamentally different kind of intelligence altogether—they exhibit unique emergent properties that can only be truly understood through direct experience and use, rather than theoretical analysis of their architecture alone.
What will happen when we effectively combine the incredible processing power and unique approach to intelligence seen in AI with the world modeling and value system advantages of human intelligence?
This combination creates unprecedented potential for information processing: AI’s extraordinary generative capabilities combined with human judgment, values, and evaluation could dramatically accelerate progress across all domains of medical practice. Scientific discovery, technological innovation, social coordination, economic development—all these depend on our ability to effectively process and apply information, and all could be transformed by this powerful synthesis. In practice, this means leveraging AI’s generative capacities to produce candidate outputs—whether research hypotheses, diagnostic suggestions, or treatment plans—which human experts then evaluate against their internal world models and value systems, selecting those that align and discarding those that do not. Yet this same combination could equally accelerate harmful outcomes if not properly directed, reinforcing the urgent need for responsible governance as well as ethical and legal safeguards.
The impact of human-AI collaboration will depend on two critical factors: the sophistication of the world models and normative frameworks that humans bring to these interactions, and the broader institutional and social structures that shape how human contribution balances AI’s raw processing power. This makes understanding and shaping the deployment of these systems perhaps the most crucial challenge we face.
In our next piece, we will explore three specific implications of this framework for law, ethics, research, and health care policy.
This post is part of a digital symposium called Innovation, Law, and Ethics in International Bioscience. To read the related posts, click here.
Acknowledgment:
This article was made possible through the generous support of the Novo Nordisk Foundation (NNF) via a grant for the scientifically independent Collaborative Research Program in Bioscience Innovation Law (Inter-CeBIL Program – Grant No. NNF23SA0087056).